Race has long been a factor in how doctors approach diagnoses— removing it has proved a challenge. Katie Palmer, Health Tech Correspondent for Stat News, joins host Krys Boyd to discuss the bias baked into medicine for decades, how it contributes to system disparities, and why the work to change it is so difficult. Her series “Embedded Bias” is written with co-author Usha Lee McFarling.
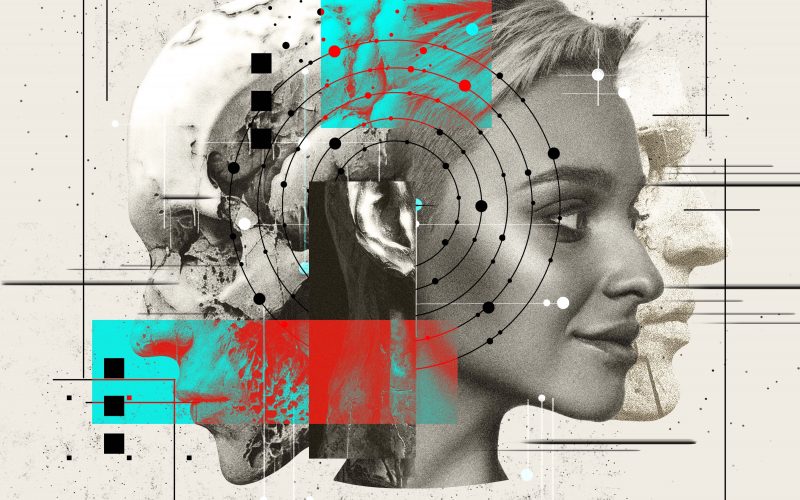
Cognitive Close up and Woman Skeleton Shutterstock